Introduction to a scale reflecting economic value of data.
We consultants are obsessed with benchmarks and scales! Why? Well, for starters benchmarking plays into the competitive nature of the typical executive who doesn’t want to be lagging or unaware, doesn’t want to miss out, and who gets into ‘fighter mode’ when their business is benchmarked to competitors.
But in reality, benchmarking is more than playing into behavioural science. Rather, executives ask for them to help determine targets and priorities, and set a course of action that will move them from a to b, or from the lower to upper quartile.
This ‘quick-win/follower’ approach results in many business pursuing ‘must-have’ projects (think of chatbots in banking). But, without a strong underpin to the overall business strategy, many data initiatives are failing.
I believe this quick-win approach typically results in jumping to solutions too quickly. After all, seeing a plot that shows, for example, ‘leaders are 5.4x more profitable than laggers, and that 78% of them have implemented chatbots’, shouldn’t automatically lead to a decision to build a chatbot!
What informs data ecosystem maturity? Let me start by stating two founding principles when it comes to data.
Data must create economic value.
Data is an ecosystem.
The first principle means that data initiatives must be aligned with business strategy, and they should strive to positively impact business outcomes — increasing profit, decreasing cost, increasing company value, decreasing risks, and so on.
The second talks to the fact that, for data initiatives to succeed, it’s rarely just about data. Rather, it’s a whole ecosystem of things that are influencing the success rate — from strategic alignment between data and business, to analytics and data itself, to data infrastructure, and to people. In short, we call this our ‘SAPI’ framework:
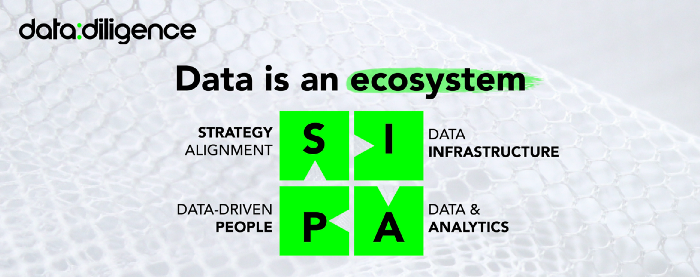
These two principles are also critically important to how we measure data-ecosystem maturity. A maturity scale must be related to economic value, not the ‘frivolities’ of data. It must also be applicable to the entire ecosystem (SAPI) and encompass all its dimensions. Furthermore, the steps on the maturity scale should be intuitive. Easy to evaluate and even self-assess. They should also be instinctively guiding companies how to move to upper levels.
So, how should we measure data ecosystem maturity? And now, without further ado, let’s look at the scale.
None: Not evident
Scattered: Sporadic pockets, lacking wide cohesion and structure
Foundational: Established at a base level across the organisation
Effective: Efficiently utilised, well-structured, and widely used
Enhanced: Ingrained and evolving to change and opportunity
Transformative: Cutting-edge approach and delivery (likely proprietary)
How does the scale work? Let’s say we are assessing a strategic factor, such as data strategy. Using our scale, a ‘back of an envelope’ assessment of the data strategy maturity could look something like this:
No vision for data = None.
Collection of arbitrary data projects = Scattered.
Strategy ensuring company has data under control and can be used for basic decision making = Foundational.
Covering how data can help target all key business issues and opportunities = Effective.
Quickly reacting to new challenges, smoothly evolving around the business needs = Enhanced.
Influencing key business decisions and even the whole business strategy = Transformative.
Applied to data representativeness — how well are data reflecting the business context of the business — the scale would indicate: No data available = None. Some factors covered, some not = Scattered. Key factors well represented in data = Foundational. Main factors represented = Effective. Important details available = Enhanced. Most of the details covered = Transformative.
Perhaps we might want to evaluate the data science toolkit. Nothing in place = None. Data scientists using basic tools based on personal preference and locally = Scattered. Basic tools for querying and analysing data available to data science team = Foundational. Solutions (products or analyses) produced by data scientists and analysts can be easily deployed and shared with other = Effective. Data science platform with enabling development, deployment, monitoring and operation of data products in place = Enhanced. Data science platform automatically suggesting and correcting solutions = Transformative.
Or how about — and let’s try something tricky — data fluency? People not aware of how data relate to their job = None. Some people using some data to make some decisions = Scattered. Most people reflecting key data when making decisions = Foundational. People comfortably making data-driven decisions = Effective. People understanding biases and limitations of data = Enhanced. People actively looking for new opportunities to leverage data to improve operations = Transformative.
And the examples could continue, but you get the point.
But why not use other data maturity scales? There are many scales for data maturity. Gartner has one. IBM too. So does BCG. And other consultancies — large and small. Even individual thought leaders like Bill Schmarzo. However, I/we believe there are some practical limitations with them.
Some are relative in nature, which makes it difficult to self-evaluate when you don’t have the benchmark.
Some consider a very specific aspect of data, making it difficult to apply the same scale to other important factors of data ecosystem (or framework).
Some are too high-level, not providing enough plasticity to enable a proper diagnosis.
Some are focusing on output, rather than impact on outcomes.
Therefore, we created a scale that meets our criteria: is easy-to-understand and intuitive, can be applied to all factors of the data ecosystem (both individually and collectively). Plus, it is tightly linked to outcomes and economic value, and provides a sequential guide through individual maturity stages.
Either way, we need to keep in mind that the value of data should be measured by business impact. Not by a scale. Rather, the scale should provide insight into where you are.
***
This article was first published on Towards Data Science https://towardsdatascience.com/measuring-data-ecosystem-maturity-62f0bf7c7143
Photo by Jukan Tateisi on Unsplash
Comments