Embracing ambiguity
- Adam Votava
- Feb 8, 2021
- 6 min read
Data literacy is more than the ability to read charts
Data is increasingly important for business success. Companies are spending greatly on data infrastructure and data professionals alike. Yet, leading opinion-makers are warning that it might not be enough.
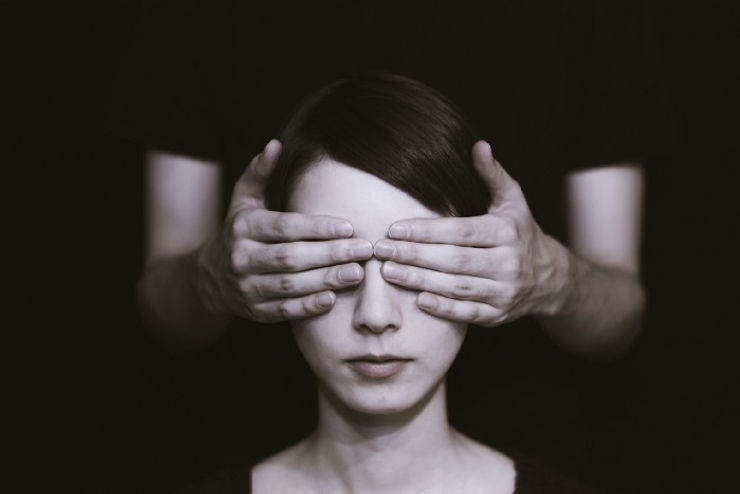
Photo by Ryoji Iwata on Unsplash
Data literacy — the ability to read, work with, analyse and communicate with data — is lacking in the majority of organisations. As a consequence, these organisations are not able to fully use the vital business resource of data to their business advantage. Leading voices are now advising on how to assess data literacy and how to boost it.
Data advocates and fanatics
There are many initiatives aiming at training people to become data literate. Most of them are doing a great job, explaining the: value of data; link between data and business; and teaching technical skills or the ability to make, read and interpret charts. This is, of course, vital as it helps to grow the number of data advocates.
However, in the much-lauded pursuit of ‘becoming data-driven’ or ‘making data-informed decisions’, people can get too enthusiastic. They start to fanatically and blindly trust data. Every single one of us has been in an emotional, heated discussion where the same data is used by both parties. But, interpreted totally differently. And, with black-and-white thinking, it is easy to label the other party as incompetent and blatantly wrong. Yet, is it that simple? While often led by good intentions, data fanatics are actually a disservice to data.
Data as a representation of the real world
In my opinion, the key to breaking this gridlock is in understanding that data is — only — a representation of the real world. Sometimes a very accurate one, sometimes less so.
Let me explain.
We were taught in school that mathematics is an exact science — a science, which demonstrates absolute precision in its results. But the same is not true of all data.
In mathematics, we abstract from the real-world objects. Whereas, in a business context, we use data to represent the real world.
An important distinction.
Meaning, the actual question we must all ask ourselves is — how well is the data reflecting the real world? Or better still, how well is the data representing the portion of the real world that matters for the problem at hand?
What to pay attention to?
This is not an easy question. The complexities of the world are enormous. Therefore, any simplification always comes with a catalogue of caveats!
Pragmatically, let me offer a few starting points.
The problem itself — how complex is it? For example: testing if the sales went up from the last year is simple. Detecting a long-term trend is a bit more complicated. Attributing the sales to individual factors can be very complex.
Also, many problems deal with randomness and uncertainty. Can the problem be solved perfectly, or do we just have to reconcile our minds (and egos) to the fact that the results will always be — to a certain extent — unpredictable?
For example, effectively allocating crews to planes given the flight schedule, aircraft assignments etc. is purely a deterministic problem — it doesn’t contain any randomness. Whereas, predicting customers’ purchasing behaviour is as uncertain as it gets.
In most cases, we cannot be paralysed by the inherent randomness and uncertainty of the problem. Rather, we must embrace the ambiguity.
“Prediction is very difficult, especially when the future is concerned” — Niels Bohr
The data. In an ideal world, we’d have all data available. In perfect quality. Reliable. And ready to support the decision-making process.
But this is never the case.
We will always have to work with a simplified model of reality. We will always have to make assumptions. And we will always have to question the quality of the data we use.
Imagine you want to predict how many visitors will visit across all your ski resorts. You can use historical data about the number of skiers. But, each resort might be using a different ticketing system so combining the data might not be easy. And you also know that the number of visitors will depend heavily on the weather. So, you could include a weather forecast in your models — but can you trust it?
Tricky. But again, that doesn’t mean we give up.
It just means there are always disclaimers when using data. Which we mustn’t hide under the proverbial carpet. Rather, we need to clearly articulate and ‘explain away’ these foibles, just as accountants do in annual financial statement notes. Afterall, this is what leads to deeper understanding and applicability and replicability.
So, strive to capture the most influential factors in your data model and maximise the data quality to increase the reliability of the final data solution.
The context. Nothing exists in a vacuum. Least of all the business problems we data scientists are challenged to solve. There will always be a bigger picture that impacts the interpretation of the results.
Imagine building a propensity model to be used to promote a product only to realise it is cannibalising your other — more profitable — products.
The real world is not a simple, or static place. New contexts emerge (often available much later in time), which can radically change how we see things. Just as historical experiences influence how we see things today. Also, as individuals and teams, we are subject to multiple cognitive (conscious and unconscious) biases. So, spend as much time on understanding the context as you do on creating the solution.
The craft. Data professionals have developed many guidelines and best practices over the years. To reduce errors in your projects I strongly encourage you to follow them. But they are not bulletproof. And often, for logical reasons, they cannot be followed to the letter. Great data science is the alchemy of art and science — with the art typically developing only after many, many mistakes and many, many use cases. This is why it’s a craft.
The models. With the increasing adoption of machine learning, we also need to understand the limitation of such techniques, despite their perceived ‘sexiness’. All come with theoretical assumptions that are rarely met in the real world. And all learn from the data available to them in the past. This training data — with all its limitations and biases — has a fundamental impact on the model performance in the real-life situation.
A credit scoring model developed in a Central European context probably won’t work in Western Europe or in Africa. Just as an NLP model trained on data from the US won’t work in Japan. And not only because of the different languages.
Unknown unknowns. Much of what I’ve discussed above touches on the known unknowns that all data scientists face. But let’s not forget the non-exhaustive list of unknown unknowns! There are a multitude of factors influencing how well the data-informed decisions will work in the real situation. So, be adaptive. And listen — to your internal compass and to your team and peers. Even listen to the fanatics, because their arguments will likely pinpoint the strengths and weaknesses in your own assumptions. Which is a neat segue to my conclusion.
Hold your opinions lightly
So far, the outlook doesn’t appear very optimistic! But that would be an unfair conclusion. Even with its imperfections, data is a highly valuable tool. A tool which, when used correctly, can have great impact on increasing a company’s profit or mitigating its risks.
It just comes with a lot of ambiguity, which shouldn’t be feared, but embraced instead. So, before you start fanatically defending the outputs of a data-product (be it a business intelligence dashboard or AI decision engine) make sure that you have considered and, where necessary, communicated the catalogue of caveats. Data can automate a lot of things. Critical thinking is not one of them.
By following a best-practice process and highlighting the assumptions you have made in the face of ambiguity (in both your approach and in your models), you will open a far richer debate with your colleagues, resulting in better analytical solutions to the business issues at hand.
Ultimately, becoming data literate means being comfortable with the ambiguity, uncertainty, randomness and the unknowns related to data.
***
As ever, I’m indefinitely grateful to Chelsea Wilkinson for patiently shaping my thoughts into a publishable format.
Opmerkingen